
Maximising Renewable Energy Investments: Forecasting and Strategic Insights
The global shift towards renewable energy is reshaping energy portfolios worldwide, with renewables like wind, solar, and hydro becoming indispensable to modern energy strategies. According to the International Energy Agency (IEA), renewables are expected to account for 90% of the increase in total global power capacity in the next five years . This rapid integration of renewables supports sustainability goals and drives energy security and economic growth.
For example, Germany, one of the leaders in renewable energy, aims to generate 80% of its electricity from renewable sources by 2030 . Similarly, corporations like Google and Apple are investing heavily in renewables to power their operations and reduce their carbon footprints.
However, the inherent variability of renewable energy sources poses significant challenges in forecasting and managing energy production. Accurate forecasting is vital for optimising investments in renewable energy. It helps stakeholders predict energy output, balance supply and demand, and make informed decisions on infrastructure investments and market strategies. For instance, effective forecasting can reduce the operational costs of wind farms by up to 20% by minimising downtime and maintenance.
Accurate forecasts not only mitigate operational risks but also enhance the financial viability of renewable energy projects. They ensure reliable and efficient integration into the grid, which is crucial for maintaining grid stability and maximising returns on investment. In this article, we explore the importance of forecasting renewable energy trends and how advanced predictive analytics and strategic insights can maximise returns on renewable energy investments. We will discuss the methodologies for accurate forecasting and their impact on investment strategies, providing a roadmap for navigating the complexities of the renewable energy market.
By understanding these dynamics, stakeholders can better align their renewable energy investments with market realities, ultimately leading to more robust and resilient energy systems.
Importance of Accurate Forecasting for Renewable Energy Sources
Accurate forecasting for renewable energy sources is crucial in managing and optimising their integration into the power grid. As renewable energy’s share in the global energy mix grows—expected to reach 30% of global electricity generation by 2025 —the ability to predict the production and availability of energy from sources like wind, solar, and hydro becomes increasingly important. The unpredictable nature of these energy sources, primarily due to weather variations, poses significant challenges for grid stability, energy pricing, and investment strategies.
Effective forecasting allows grid operators to predict fluctuations in energy supply, enabling better scheduling and balancing of conventional power plants, and reducing reliance on fossil fuels during renewable energy dips. This predictive capability not only enhances the stability of the power grid but also helps in maintaining a consistent energy supply to meet demand, thus reducing the risk of blackouts and ensuring a more resilient energy infrastructure.
Methods and Tools Used for Forecasting
Several sophisticated methods and tools are employed to forecast renewable energy production accurately:
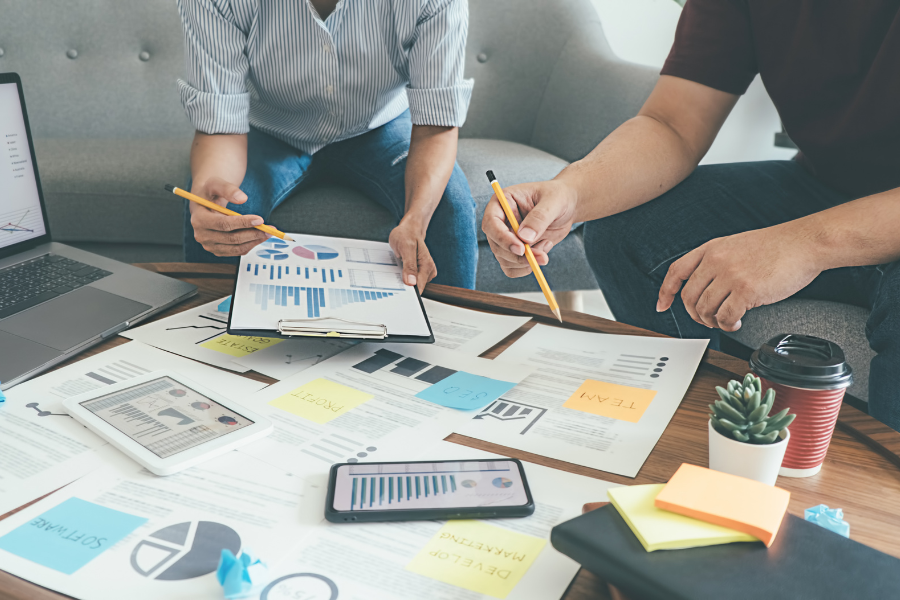
- Numerical Weather Prediction (NWP): NWP models, such as those used by the European Centre for Medium-Range Weather Forecasts (ECMWF), provide detailed weather forecasts essential for predicting wind and solar energy output. These models use data assimilation techniques and complex algorithms to simulate atmospheric conditions and predict weather patterns that impact renewable energy generation.
- Statistical Models: Techniques like time-series analysis and regression models leverage historical weather data and energy production patterns to forecast future energy outputs. Machine learning algorithms, such as neural networks and support vector machines, enhance these models’ accuracy by identifying complex, non-linear relationships between variables.
- Remote Sensing and Satellite Data: Remote sensing technologies, including LiDAR and satellite imagery, offer real-time data on cloud cover, wind speeds, and solar irradiance. These tools improve the precision of short-term forecasts by providing up-to-date information on the environmental factors affecting renewable energy sources.
- Energy Management Systems (EMS): Modern EMS incorporate real-time data monitoring and advanced analytics to optimise the operation of renewable energy assets. They integrate forecasting models with operational data, enabling dynamic adjustments to energy production and distribution strategies.
Case Study: Implementing Accurate Forecasting for Improved Predictions
Case Study: Increasing Operational Efficiency with Accurate Forecasting in Geothermal Energy
GeoPower International: GeoPower International, specialising in geothermal energy, adopted advanced forecasting techniques to enhance their energy extraction processes. Leveraging seismic data, thermal readings, and predictive models, GeoPower accurately forecasted energy yields from geothermal wells. This approach enabled optimal drilling schedules and energy extraction methods, resulting in a 22% increase in energy production and a 17% reduction in operational costs.
Results of Effective Forecasting
The benefits of accurate forecasting in renewable energy investments are manifold:
- Cost Savings: Effective forecasting minimises operational costs by reducing the need for backup power and enhancing the scheduling of maintenance activities. For instance, the Danish wind energy sector saved approximately $30 million annually through improved wind power forecasting.
- Increased Efficiency: Accurate predictions allow for better integration of renewable energy into the grid, reducing the need for conventional power sources and optimising the use of renewable energy. This leads to more efficient energy systems and lower emissions.
- Enhanced Investment Confidence: Investors gain confidence in renewable energy projects with reliable forecasts, as they provide a clearer picture of expected returns and reduce financial risks associated with energy production variability.
Challenges in Renewable Energy Forecasting
Despite the advancements, several challenges remain:
- Data Accuracy and Availability: Reliable forecasting depends on accurate, high-resolution weather and operational data. Inadequate data quality or gaps in data can significantly affect forecast accuracy.
- Model Complexity: Forecasting models need to account for numerous variables and their interactions, which can be complex and computationally intensive. Simplifying these models without losing accuracy is a major challenge.
- Integration with Grid Operations: Integrating forecasting tools with existing grid management systems requires overcoming technical and regulatory hurdles, ensuring compatibility and real-time responsiveness.
- Weather Variability: The inherent unpredictability of weather conditions, especially in regions with volatile climates, poses a continuous challenge for accurate forecasting. Enhancing model robustness to handle such variability is crucial for reliable energy predictions.
Accurate forecasting plays a pivotal role in maximising the benefits of renewable energy investments. By leveraging advanced methods and tools, stakeholders can optimise energy production, enhance grid stability, and achieve significant cost savings. While challenges persist, ongoing innovations and improved data integration are paving the way for more reliable and efficient renewable energy systems. As the energy landscape continues to evolve, embracing accurate forecasting will be key to harnessing the full potential of renewable energy sources, driving a sustainable and resilient energy future.
Challenges and Strategies in Forecasting Renewable Energy and Managing Diversified Energy Portfolios
Common Challenges in Forecasting Renewable Energy
Intermittency and Weather Variability
One of the primary challenges in forecasting renewable energy is the intermittency of sources like wind and solar. Unlike fossil fuel plants, renewable energy production can fluctuate significantly based on weather conditions. For example, wind speeds can vary hourly, and solar power generation depends on sunlight availability, which can be affected by cloud cover and seasonal changes.
The intermittency of renewables poses a problem for grid stability and energy supply reliability. For instance, a sudden drop in wind speed can cause wind farms to produce less energy than anticipated, leading to potential power shortages if backup sources aren’t available. Similarly, solar energy production can be unpredictable on cloudy days, impacting the overall energy output.
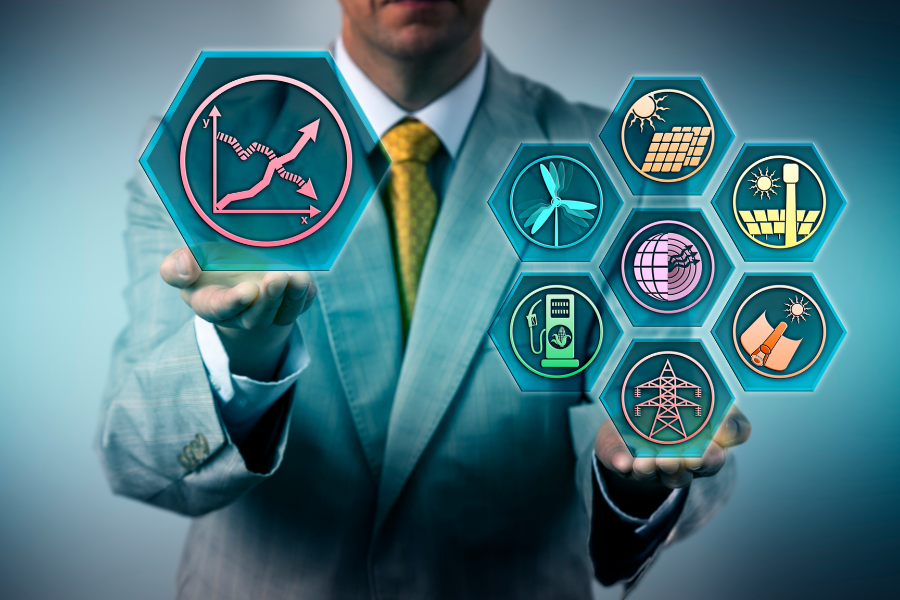
Data Accuracy and Quality
Accurate forecasting relies heavily on high-quality, real-time data. However, obtaining precise meteorological and operational data can be challenging. Weather data, essential for predicting energy outputs, often varies in resolution and accuracy, depending on the source. Additionally, operational data from energy plants may suffer from inconsistencies or gaps, affecting the reliability of forecasts.
For example, a study from the National Renewable Energy Laboratory (NREL) highlighted that improved wind speed predictions could reduce forecast errors by up to 30%, but achieving this requires high-resolution data, which isn’t always available or cost-effective to obtain.
Model Complexity
Renewable energy forecasting models need to account for numerous variables, including weather patterns, geographic conditions, and plant performance. This complexity requires sophisticated algorithms and computational resources. Balancing model accuracy with computational efficiency is a significant challenge, as overly complex models can be slow and costly to run, while simpler models may lack precision.
Integration with Grid Operations
Integrating renewable energy forecasts with existing grid management systems is another hurdle. Grid operators need to incorporate forecast data into their decision-making processes to balance supply and demand effectively. This integration requires overcoming technical barriers and ensuring compatibility with existing infrastructure.
Strategies to Overcome Forecasting Challenges
Advanced Forecasting Techniques
To address the challenge of intermittency, advanced forecasting techniques such as Numerical Weather Prediction (NWP) models and machine learning algorithms are employed. NWP models simulate atmospheric conditions using mathematical equations, providing detailed weather forecasts that can predict wind speeds and solar irradiance with greater accuracy. For example, the European Centre for Medium-Range Weather Forecasts (ECMWF) offers high-resolution weather predictions that can significantly enhance the accuracy of renewable energy forecasts.
Machine learning algorithms can analyse historical data and identify patterns that traditional statistical methods might miss. These algorithms improve over time as they process more data, leading to better forecast accuracy. For instance, Google’s DeepMind AI improved the energy output prediction for its wind farms by 20% by using machine learning to analyse historical weather data.
Improved Data Collection and Quality
Enhancing data accuracy involves investing in better data collection technologies, such as remote sensing, LiDAR, and satellite imagery. These tools provide high-resolution, real-time data on environmental conditions affecting renewable energy production. Implementing rigorous data validation and cleansing processes can also improve data quality, ensuring more reliable forecasts.
Hybrid Models
Combining multiple forecasting models can also mitigate the limitations of individual models. Hybrid models integrate different forecasting techniques, such as statistical models with machine learning, to balance their strengths and weaknesses. This approach can provide more robust and accurate predictions.
Grid Integration and Flexibility
To integrate forecasts with grid operations effectively, developing flexible grid systems that can quickly respond to changes in energy production is crucial. This includes investing in smart grid technologies and demand response programs that adjust energy usage based on forecast data. For example, California’s Independent System Operator (CAISO) uses advanced grid management systems that incorporate real-time renewable energy forecasts, allowing for better balancing of supply and demand.
Managing Portfolio with Renewable Energy
Importance of Integrating Renewables
Integrating renewables into the energy portfolio is essential for achieving sustainability goals and reducing carbon emissions. Renewable energy sources, such as wind, solar, and hydro, offer clean alternatives to fossil fuels and help diversify energy supply, enhancing energy security and reducing reliance on non-renewable resources.
For instance, Denmark generates over 50% of its electricity from wind energy, significantly reducing its carbon footprint and reliance on imported fossil fuels. This shift has also positioned Denmark as a leader in renewable energy technology and innovation.
Strategies for Managing a Diversified Energy Portfolio
Effectively managing a diversified energy portfolio involves balancing renewable and conventional energy sources to ensure reliable and cost-effective energy supply. Key strategies include:
- Energy Storage Solutions: Integrating energy storage systems, like batteries, can store excess renewable energy produced during peak times for use during low production periods. This helps balance supply and demand, reducing the impact of intermittency.
- Demand Response Programs: Implementing demand response strategies allows energy consumers to adjust their usage based on energy availability, helping to stabilise the grid during periods of high demand or low renewable energy production.
- Dynamic Pricing Models: Adopting dynamic pricing models that reflect real-time energy costs can incentivize consumers to use energy during periods of high renewable production, aligning consumption with availability.
Role of Forecasting in Portfolio Management
Accurate forecasting plays a pivotal role in optimising portfolio management by providing insights into expected energy production and demand patterns. These forecasts enable better planning and decision-making, reducing operational risks and enhancing the financial performance of renewable energy investments. For example, Germany’s use of sophisticated forecasting tools for its wind and solar farms has improved energy scheduling and grid management, contributing to higher integration of renewables and reduced curtailment rates.
Accurate forecasting and effective portfolio management are essential for maximising the benefits of renewable energy investments. By leveraging advanced forecasting techniques, improving data quality, and integrating flexible grid systems, stakeholders can overcome the challenges associated with renewable energy production and ensure a more sustainable and resilient energy future. As the energy landscape continues to evolve, embracing these strategies will be key to harnessing the full potential of renewable energy sources.
Conclusion
Accurate forecasting is crucial for maximising investments in renewable energy by ensuring efficient integration and management of these variable sources. Leveraging advanced tools and techniques helps address challenges like intermittency and data accuracy, leading to more reliable energy production and cost savings. As forecasting technologies continue to advance, they will play an even greater role in optimising renewable energy portfolios, driving the transition toward a more sustainable and resilient energy future.